- Los agentes de IA van desde simples sistemas reflejos que reaccionan a las entradas actuales a sofisticados agentes que planifican, aprenden y coordinan tareas complejas en diversos ámbitos.
- Los agentes reflejos simples actúan exclusivamente en función de las condiciones inmediatas, mientras que los agentes reflejos basados en modelos mejoran la toma de decisiones utilizando modelos internos para seguir y predecir los cambios del entorno.
- Los sistemas multiagente presentan múltiples agentes de IA que trabajan juntos o compiten entre sí, lo que permite aplicaciones avanzadas como la coordinación de coches autoconducidos en el tráfico o la gestión de intrincadas cadenas de suministro.
Los agentes de IA se han disparado en los últimos años. Y con su compleja tecnología y capacidades, hoy en día hay muchos tipos diferentes de agentes de IA.
Un agente de IA es un software que realiza tareas. A diferencia de un chatbot estándar, puede realizar acciones en nombre de un usuario.
Hay una amplia gama de agentes de IA, desde termómetros inteligentes y coches autoconducidos hasta agentes con interfaces de chat. Todos estos casos de uso entran dentro de una de las siete categorías principales de agentes de IA. En este artículo, compartiré los 7 tipos principales de agentes de IA y algunos ejemplos del mundo real de agentes de IA.
1. Agentes reflejos simples
Un agente reflejo simple es un sistema de IA que toma decisiones basándose únicamente en la información actual de su entorno.
Utiliza un conjunto de reglas de condición-acción para asignar entradas observadas a respuestas específicas. Cuando detecta un determinado estado en el entorno, ejecuta la regla correspondiente.
No tiene memoria ni modelo interno del mundo, por lo que sólo puede funcionar eficazmente en entornos totalmente observables en los que cada decisión puede tomarse basándose únicamente en la información actual.
Ejemplos de agentes reflejos simples
- Un termostato que enciende la calefacción si hace demasiado frío
- Un robot que gira al chocar contra una pared (hola, Roomba con un gato encima)
- Un chatbot básico que responde "¡Hola!" cuando un usuario dice "Hola".
.webp)
2. Agentes reflejos basados en modelos
Un agente reflejo basado en un modelo es un agente de IA que toma decisiones basándose tanto en la entrada actual como en un modelo interno del mundo.
A diferencia de los agentes reflejos simples, este tipo de agente realiza un seguimiento del estado del entorno a lo largo del tiempo. Utiliza un modelo -esencialmente, información almacenada sobre cómo funciona el mundo- para rellenar huecos cuando el entorno no es totalmente observable.
Cuando recibe una nueva entrada, actualiza su estado interno, consulta sus reglas de condición-acción y elige la mejor respuesta basándose tanto en la percepción actual como en lo que sabe de interacciones anteriores.
Ejemplos de agentes reflejos basados en modelos
- Un robot aspirador que recuerda la distribución de una habitación y evita las zonas que ya ha limpiado.
- Un agenteLLM que continúa una conversación sin perder de vista las entradas anteriores del usuario.
- Una IA de juego que reacciona no sólo a lo que ve, sino también a lo que sabe desde antes en el partido.
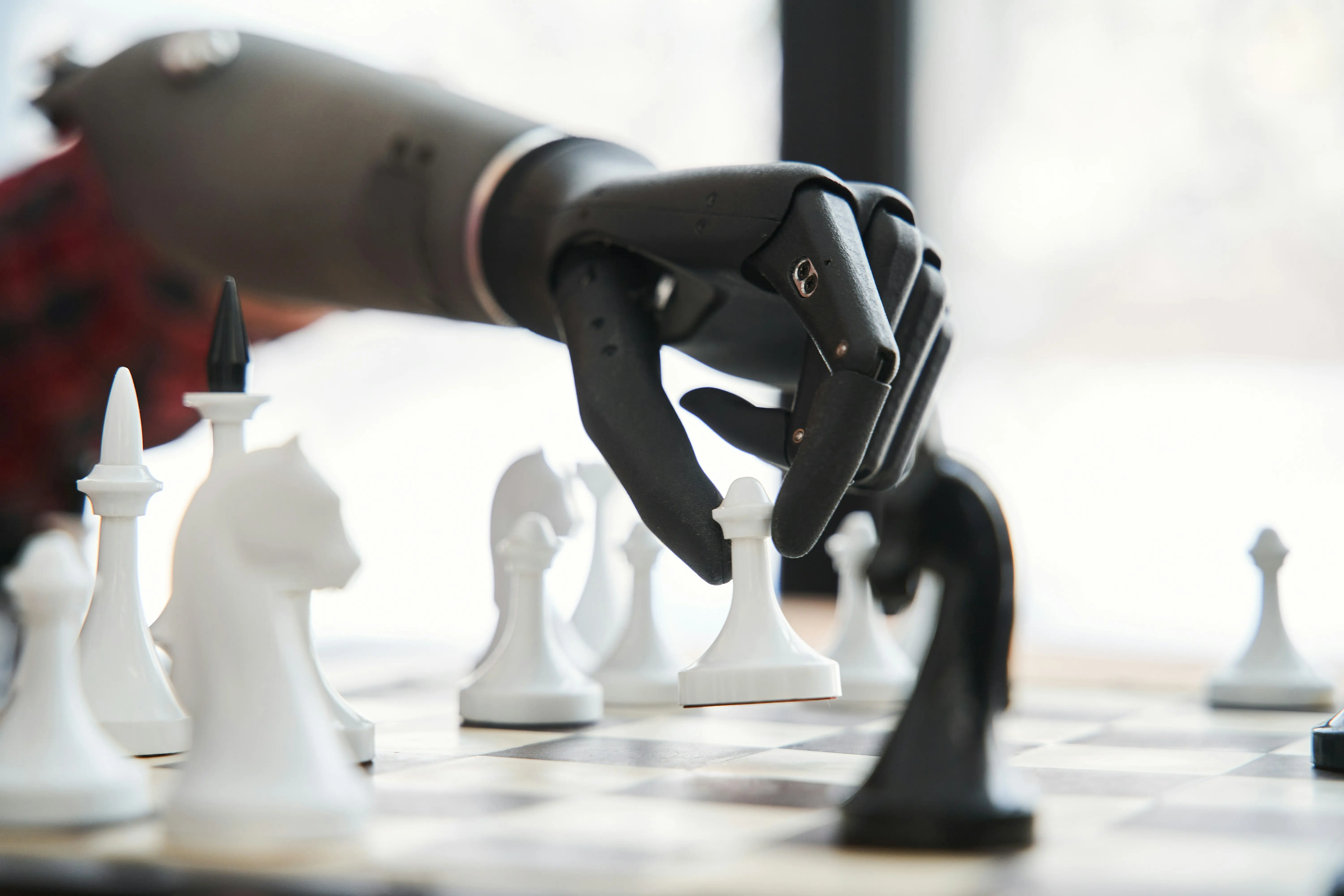
3. Agentes de aprendizaje
Un agente que aprende es un agente de IA que mejora su rendimiento con el tiempo aprendiendo de sus experiencias.
Tiene cuatro componentes principales: un elemento de aprendizaje, un elemento de rendimiento, un crítico y un generador de problemas.
El elemento de rendimiento elige las acciones, mientras que el elemento de aprendizaje ajusta su comportamiento basándose en la retroalimentación. El crítico evalúa el resultado de las acciones utilizando una norma predefinida, y el generador de problemas sugiere nuevas acciones a probar para mejorar el aprendizaje.
Esta estructura permite al agente adaptarse a los cambios, perfeccionar las estrategias y operar con eficacia incluso en entornos desconocidos.
Ejemplos de agentes de aprendizaje
- Un agente de inteligencia artificial para criptomonedas que ajusta las estrategias de negociación en función de la evolución del mercado
- Un motor de recomendación que sugiere mejor los productos en función del comportamiento del usuario.
- Un chatbot sanitario que aprende de las interacciones de los pacientes para mejorar la precisión del triaje

4. Agentes basados en la utilidad
Un agente basado en la utilidad es un agente de IA que elige acciones basándose en qué resultado se espera que proporcione el mayor valor global o "utilidad".
En lugar de limitarse a alcanzar un objetivo, este agente evalúa diferentes resultados posibles y selecciona el que maximiza una función de utilidad predefinida.
Esto le permite manejar situaciones en las que hay varias formas de alcanzar un objetivo o en las que hay que hacer concesiones. Requiere la capacidad de comparar opciones, predecir consecuencias y clasificar resultados en función de preferencias o prioridades.
Ejemplos de agentes basados en la utilidad
- Un chatbot para ventas que prioriza los clientes potenciales en función de la probabilidad de conversión
- Un robot que equilibra riesgo y rentabilidad para maximizar las ganancias a largo plazo
- Un chatbot empresarial que programa reuniones para minimizar los conflictos y maximizar la comodidad.
5. Agentes jerárquicos
Un agente jerárquico es un agente de IA que organiza su proceso de toma de decisiones en múltiples capas o niveles, con niveles superiores que manejan objetivos abstractos y niveles inferiores que gestionan acciones específicas.
Este agente divide las tareas complejas en subtareas más pequeñas, y cada nivel de la jerarquía es responsable de un ámbito distinto de la toma de decisiones.
Las capas de alto nivel pueden planificar estrategias a largo plazo, mientras que las capas inferiores gestionan los datos inmediatos de los sensores y las respuestas en tiempo real. La comunicación fluye entre capas, lo que permite al agente coordinar objetivos amplios con una ejecución detallada.
Esta estructura facilita la gestión de la complejidad y el comportamiento a escala en diferentes plazos o prioridades.
Ejemplos de agentes jerárquicos
- En la fabricación, un agente de alto nivel planifica el proceso de ensamblaje, mientras que los niveles inferiores controlan los brazos robóticos y los tiempos.
- En una fábrica inteligente, diferentes niveles gestionan los programas de producción, la coordinación de las máquinas y las operaciones físicas.
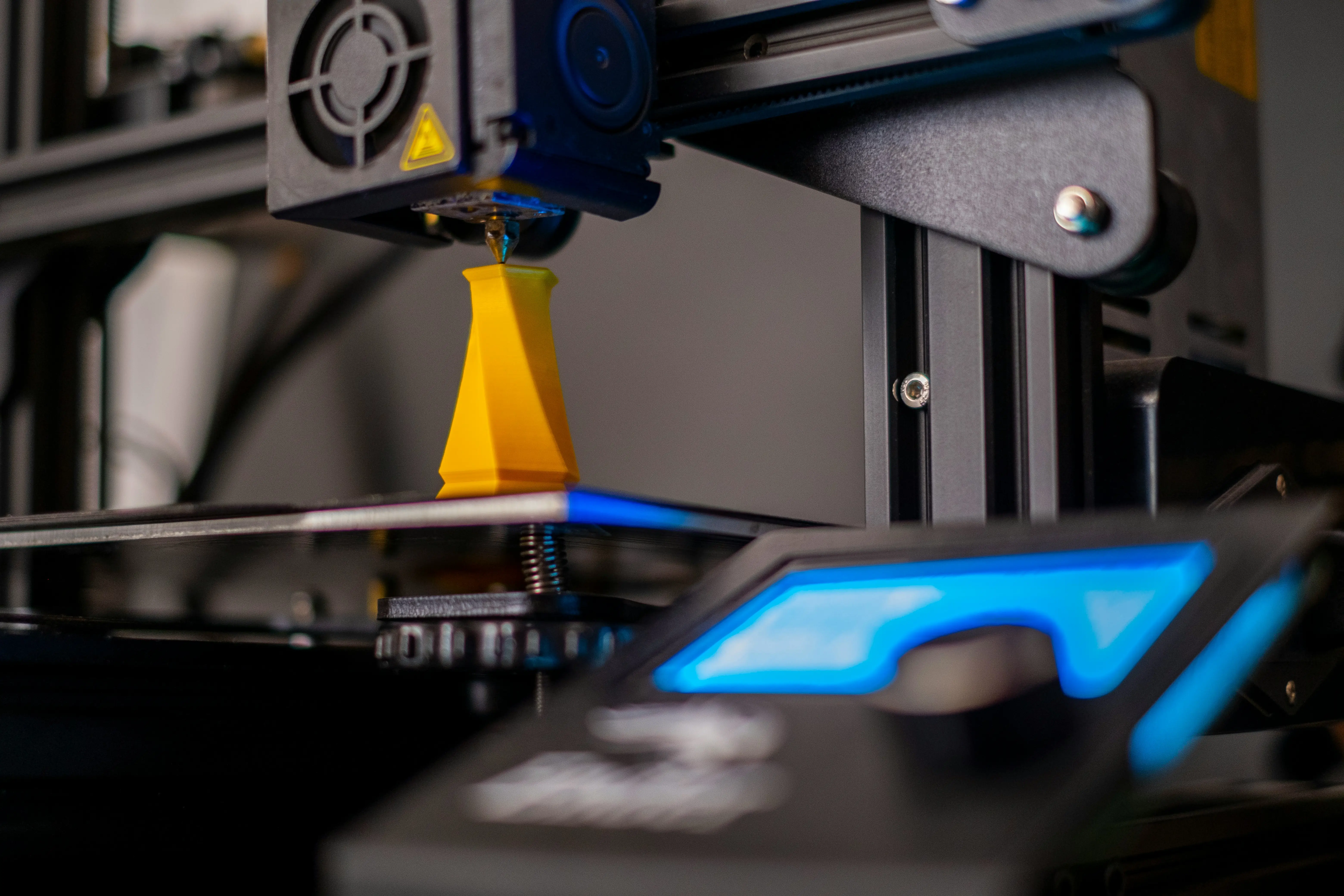
6. Agentes basados en objetivos
Un agente basado en objetivos es un agente de IA que toma decisiones evaluando qué acciones le ayudarán a alcanzar un objetivo específico.
El agente recibe uno o varios objetivos, es decir, los resultados deseados. Utiliza algoritmos de búsqueda o planificación para explorar posibles secuencias de acciones y, a continuación, selecciona las que tienen más probabilidades de conducir al objetivo.
A diferencia de los agentes reflejos, no se limita a reaccionar, sino que razona sobre las consecuencias futuras antes de actuar. Esto lo hace más flexible y capaz en entornos dinámicos o desconocidos, pero también más exigente desde el punto de vista computacional.
Ejemplos de agentes basados en objetivos
- Un sistema de navegación que calcula la mejor ruta para llegar a un destino
- Una inteligencia artificial que busca movimientos que lleven a completar el puzzle.
- Un brazo robótico que planifica una secuencia de movimientos para ensamblar con éxito un producto.
7. Sistemas multiagente (MAS)
Por último, pero no por ello menos importante: el sistema multiagente.
Un sistema multiagente (SAM) es un sistema compuesto por múltiples agentes IA que interactúan y trabajan juntos (o a veces compiten) para alcanzar objetivos individuales o compartidos.
Cada agente del sistema funciona de forma independiente, con sus propias capacidades, objetivos y percepción del entorno.
Estos agentes se comunican y coordinan, ya sea directamente a través de mensajes o indirectamente mediante la observación de los cambios en el entorno. El sistema en su conjunto puede resolver problemas demasiado complejos o distribuidos como para que un solo agente se ocupe de ellos.
Los sistemas multiagente pueden ser cooperativos, competitivos o una mezcla de ambos, dependiendo del diseño y los objetivos.
Ejemplos de sistemas multiagente
- Vehículos autónomos se coordinan en un cruce para evitar colisiones
- Un conjunto de bots financieros gestiona la facturación, la detección de fraudes y la elaboración de informes mediante la automatización del flujo de trabajo con IA.
- Un sistema de cadena de suministro en el que diferentes agentes gestionan las existencias, los envíos y la previsión de la demanda.

Crea agentes de IA personalizados
No es difícil crear un agente de IA personalizado, y puedes hacerlo gratis.
Botpress ofrece un constructor de flujo visual de arrastrar y soltar, seguridad de nivel empresarial, una amplia biblioteca educativa y una comunidadDiscord activa de más de 20.000 creadores de bots.
Nuestra plataforma extensible le permite crear cualquier chatbot personalizado con cualquier integración personalizada, y nuestro Hub integración está repleto de conectores prediseñados con los canales más importantes.
Empieza a construir hoy mismo. Es gratis.
Preguntas frecuentes
¿Cuáles son los 7 tipos de agente de IA?
Los 7 tipos son: agentes reflejos simples, agentes reflejos basados en modelos, agentes basados en objetivos, agentes basados en utilidades, agentes de aprendizaje, agentes jerárquicos y sistemas multiagente.
¿Es ChatGPT un agente de IA?
Sí, ChatGPT puede considerarse un agente de IA: recibe información, la procesa y genera respuestas, a menudo con un enfoque basado en objetivos o utilidades, dependiendo de cómo se utilice.
¿Qué son los agentes inteligentes y cómo actúan en los entornos digitales?
Los agentes inteligentes son entidades diseñadas para actuar en diversos entornos digitales. Recogen conocimientos de su entorno, evalúan la situación actual y ejecutan acciones para alcanzar objetivos predefinidos. Su rendimiento se ve influido por las acciones externas que realizan en entornos observables.
¿Qué papel desempeña la inteligencia artificial en la funcionalidad de los agentes?
La Inteligencia Artificial permite a los agentes inteligentes aprender, razonar y adaptarse. Los agentes utilizan la IA para mejorar su base de conocimientos, lo que les permite tomar decisiones más sofisticadas en diversos entornos.
¿Qué constituye la base de conocimientos de los agentes inteligentes?
El conocimiento de los agentes inteligentes abarca información sobre el entorno, reglas predefinidas y una comprensión fundamental de la situación actual. Este conocimiento constituye la base de sus procesos de toma de decisiones.
¿Qué es el elemento de rendimiento en el contexto de los agentes inteligentes?
El elemento de rendimiento de los agentes inteligentes se refiere a su capacidad para alcanzar objetivos y tomar decisiones que optimicen sus acciones en un entorno determinado. Es un componente crucial que determina la eficiencia y eficacia del agente.
¿Pueden los agentes operar en estructuras jerárquicas?
Sí, los agentes jerárquicos son un tipo de agente inteligente que opera en niveles estructurados. Los agentes de alto nivel supervisan la toma de decisiones generales, mientras que los agentes de nivel inferior se encargan de tareas específicas dentro de un marco más amplio. Esta estructura jerárquica permite un funcionamiento eficaz en entornos complejos.
¿Operan los agentes inteligentes con inteligencia limitada?
Sí, muchos agentes inteligentes operan con inteligencia limitada, lo que significa que tienen un ámbito definido de conocimientos y capacidades. Esta limitación les ayuda a centrarse en tareas y entornos específicos en los que su experiencia es más relevante.