
近年来,人工智能代理呈爆炸式增长。由于其技术和功能复杂,如今有很多不同类型的人工智能代理。
人工智能代理是一种执行任务的软件。与标准聊天机器人不同,它可以代表用户采取行动。
There's a wide range of AI agents, from smart thermometers and self-driving cars, to agents with chat interfaces. All of these use cases fall into one of the seven main categories of AI agents. In this article, I'll share the 7 main types of AI agent and some real-world examples of AI agents.
1.简单反射剂
A simple reflex agent is an AI system that makes decisions based only on the current input from its environment.
It uses a set of condition-action rules to map observed inputs to specific responses. When it detects a certain state in the environment, it executes the corresponding rule.
It has no memory or internal model of the world — so it can only operate effectively in fully observable environments where every decision can be made based on the current input alone.
Examples of Simple Reflex Agents
- A thermostat that turns on the heat if it’s too cold
- A robot that turns when it hits a wall (hello, Roomba with a cat on top)
- A basic chatbot that replies “Hello!” when a user says “Hi”
.webp)
2.基于模型的反射代理
A model-based reflex agent is an AI agent that makes decisions based on both the current input and an internal model of the world.
Unlike simple reflex agents, this type keeps track of the environment’s state over time. It uses a model — essentially, stored information about how the world works — to fill in gaps when the environment isn’t fully observable.
When it receives a new input, it updates its internal state, consults its condition-action rules, and chooses the best response based on both the current percept and what it knows from previous interactions.
Examples of Model-Based Reflex Agents
- A robot vacuum that remembers the layout of a room and avoids areas it has already cleaned
- An LLM agent that continues a conversation while keeping track of past user inputs
- A game AI that reacts not only to what it sees but also to what it knows from earlier in the match
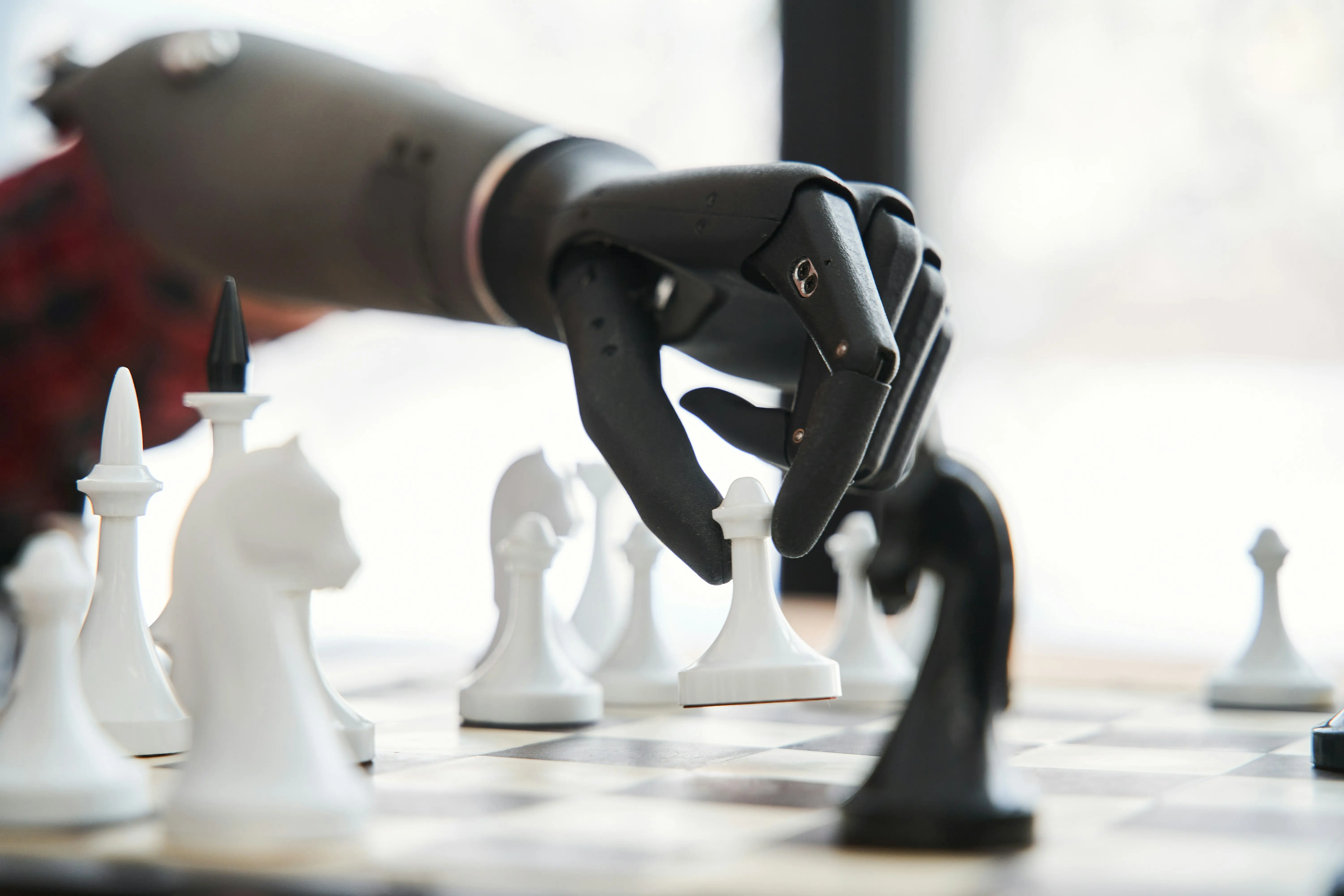
3.学习型代理
A learning agent is an AI agent that improves its performance over time by learning from its experiences.
It has four main components: a learning element, a performance element, a critic, and a problem generator.
The performance element chooses actions, while the learning element adjusts its behavior based on feedback. The critic evaluates the outcome of actions using a predefined standard, and the problem generator suggests new actions to try for better learning.
This structure allows the agent to adapt to changes, refine strategies, and operate effectively even in unfamiliar environments.
Examples of Learning Agents
- A crypto AI agent that adjusts trading strategies based on market performance
- A recommendation engine that gets better at suggesting products based on user behavior
- A healthcare chatbot that learns from patient interactions to improve triage accuracy

4.基于效用的代理
A utility-based agent is an AI agent that chooses actions based on which outcome is expected to provide the highest overall value or “utility.”
Rather than just aiming to achieve a goal, this agent evaluates different possible outcomes and selects the one that maximizes a predefined utility function.
This allows it to handle situations where there are multiple ways to reach a goal, or where trade-offs must be made. It requires the ability to compare options, predict consequences, and rank outcomes based on preferences or priorities.
Examples of Utility-Based Agents
- A chatbot for sales that prioritizes leads based on likelihood to convert
- A stock trading bot that balances risk and return to maximize long-term gains
- A business chatbot that schedules meetings to minimize conflicts and maximize convenience
5.分层代理
A hierarchical agent is an AI agent that organizes its decision-making process into multiple layers or levels, with higher levels handling abstract goals and lower levels managing specific actions.
This agent breaks complex tasks into smaller sub-tasks, with each level of the hierarchy responsible for a different scope of decision-making.
High-level layers may plan long-term strategies, while lower layers handle immediate sensor data and real-time responses. Communication flows between layers, allowing the agent to coordinate broad objectives with detailed execution.
This structure makes it easier to manage complexity and scale behavior across different time frames or priorities.
Examples of Hierarchical Agents
- In manufacturing, a high-level agent plans the assembly process while lower levels control robotic arms and timing
- In a smart factory, different layers manage production schedules, machine coordination, and physical operations
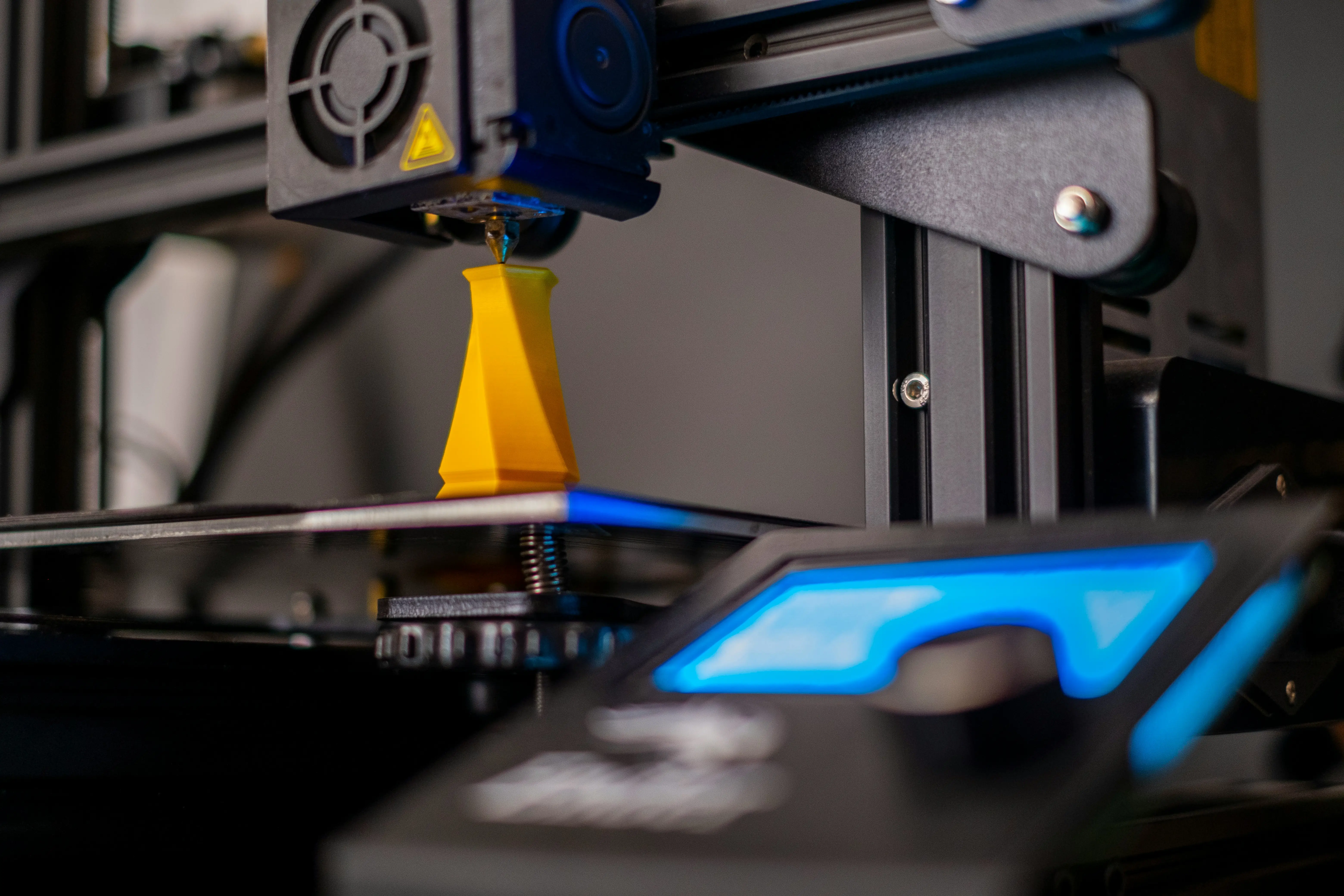
6. Goal-Based Agents
A goal-based agent is an AI agent that makes decisions by evaluating which actions will help it achieve a specific goal.
The agent is given one or more goals — desired outcomes it wants to reach. It uses search or planning algorithms to explore possible sequences of actions, then selects the ones that are most likely to lead to the goal.
Unlike reflex agents, it doesn't just react — it reasons about future consequences before acting. This makes it more flexible and capable in dynamic or unfamiliar environments, but also more computationally demanding.
Examples of Goal-Based Agents
- A navigation system that calculates the best route to a destination
- A puzzle-solving AI that searches for moves that will lead to a completed puzzle
- A robotic arm that plans a sequence of motions to successfully assemble a product
7. Multi-Agent Systems (MAS)
Last but not least: the multi-agent system.
A multi-agent system (MAS) is a system composed of multiple interacting AI agents that work together (or sometimes compete) to accomplish individual or shared objectives.
Each agent in the system operates independently, with its own capabilities, goals, and perception of the environment.
These agents communicate and coordinate — either directly through messages or indirectly by observing changes in the environment. The system as a whole can solve problems that are too complex or distributed for a single agent to handle.
Multi-agent systems can be cooperative, competitive, or a mix of both, depending on the design and goals.
Examples of Multi-Agent Systems
- Autonomous vehicles coordinating at an intersection to avoid collisions
- A set of finance bots manages invoicing, fraud detection, and reporting through AI workflow automation
- A supply chain system where different agents manage inventory, shipping, and demand forecasting

构建自定义人工智能代理
It's not difficult to build a customized AI agent - and you can do it for free.
Botpress 提供拖放式可视化流程生成器、企业级安全性、丰富的教育资料库以及由 20,000 多名机器人构建者组成的活跃Discord 社区。
我们的平台具有可扩展性,这意味着您可以使用任何自定义集成构建任何自定义聊天机器人--我们的集成Hub预置了大量连接最大渠道的连接器。
今天就开始建设。它是免费的。
常见问题
Is ChatGPT an AI agent?
Yes, ChatGPT can be considered an AI agent — it receives input, processes it, and generates responses, often using a goal or utility-driven approach depending on how it’s deployed.
What are the 7 types of AI agent?
The 7 types are: simple reflex agents, model-based reflex agents, goal-based agents, utility-based agents, learning agents, hierarchical agents, and multi-agent systems.
什么是智能代理,它们如何在数字环境中运行?
智能代理是设计用于在各种数字环境中行动的实体。它们从周围环境中收集知识,评估当前形势,并执行行动以实现预定目标。它们的性能受其在可观察环境中所采取的外部行动的影响。
人工智能如何在代理功能中发挥作用?
人工智能赋予智能代理学习、推理和适应的能力。代理利用人工智能来增强自己的知识库,从而在各种环境中做出更复杂的决策。
什么是智能代理的知识库?
智能代理的知识包括环境信息、预定义规则和对当前情况的基本了解。这些知识构成了它们决策过程的基础。
什么是智能代理的性能要素?
智能代理的性能要素是指它们在特定环境中实现目标和做出优化行动决策的能力。它是决定代理效率和效力的关键要素。
代理人能否在等级结构中运作?
是的,分层代理是一种按结构层次运行的智能代理。高级代理负责总体决策,而低级代理则在更广泛的框架内处理具体任务。这种分层结构可以在复杂的环境中高效运行。
智能代理是否以有限的智能运行?
是的,许多智能代理都是在有限智能的情况下运行的,这意味着它们有明确的知识和能力范围。这种限制有助于它们专注于特定的任务和环境,因为在这些任务和环境中,它们的专业知识最为相关。
目录
分享到