- Les agents d'intelligence artificielle vont de simples systèmes réflexes réagissant aux données actuelles à des agents sophistiqués qui planifient, apprennent et coordonnent des tâches complexes dans divers domaines.
- Les agents réflexes simples agissent uniquement en fonction des conditions immédiates, tandis que les agents réflexes basés sur des modèles améliorent la prise de décision en utilisant des modèles internes pour suivre et prédire les changements environnementaux.
- Les systèmes multi-agents se composent de plusieurs agents d'intelligence artificielle qui travaillent ensemble ou sont en concurrence, ce qui permet des applications avancées telles que la coordination des voitures autonomes dans le trafic ou la gestion de chaînes d'approvisionnement complexes.
Les agents d'intelligence artificielle ont explosé ces dernières années. La complexité de leur technologie et de leurs capacités fait qu'il existe aujourd'hui de nombreux types d'agents d'intelligence artificielle.
Un agent d'intelligence artificielle est un logiciel qui exécute des tâches. Contrairement à un chatbot standard, il peut prendre des mesures au nom d'un utilisateur.
Il existe un large éventail d'agents d'intelligence artificielle, allant des thermomètres intelligents aux voitures autonomes, en passant par les agents dotés d'interfaces de discussion. Tous ces cas d'utilisation entrent dans l'une des sept catégories principales d'agents d'IA. Dans cet article, je vais vous présenter les sept principaux types d'agents d'IA et quelques exemples concrets d'agents d'IA.
1. Agents réflexes simples
Un agent réflexe simple est un système d'intelligence artificielle qui prend des décisions en se basant uniquement sur les données actuelles de son environnement.
Il utilise un ensemble de règles de condition-action pour faire correspondre les entrées observées à des réponses spécifiques. Lorsqu'il détecte un certain état dans l'environnement, il exécute la règle correspondante.
Il n'a pas de mémoire ni de modèle interne du monde. Il ne peut donc fonctionner efficacement que dans des environnements entièrement observables où chaque décision peut être prise sur la seule base des données actuelles.
Exemples d'agents réflexes simples
- Un thermostat qui allume le chauffage s'il fait trop froid
- Un robot qui se retourne lorsqu'il heurte un mur (bonjour, Roomba avec un chat sur le dessus)
- Un chatbot basique qui répond "Hello !" lorsqu'un utilisateur dit "Hi"
.webp)
2. Agents réflexes basés sur un modèle
Un agent réflexe basé sur un modèle est un agent d'intelligence artificielle qui prend des décisions basées à la fois sur les données actuelles et sur un modèle interne du monde.
Contrairement aux agents réflexes simples, ce type d'agent suit l'état de l'environnement au fil du temps. Il utilise un modèle - essentiellement des informations stockées sur le fonctionnement du monde - pour combler les lacunes lorsque l'environnement n'est pas entièrement observable.
Lorsqu'il reçoit une nouvelle information, il met à jour son état interne, consulte ses règles de condition-action et choisit la meilleure réponse en fonction du percept actuel et de ce qu'il sait des interactions précédentes.
Exemples d'agents réflexes basés sur des modèles
- Un aspirateur robot qui se souvient de la disposition d'une pièce et évite les zones qu'il a déjà nettoyées
- Un agentLLM qui poursuit une conversation tout en gardant la trace des interventions antérieures de l'utilisateur.
- Une IA de jeu qui réagit non seulement à ce qu'elle voit, mais aussi à ce qu'elle sait depuis le début du match.
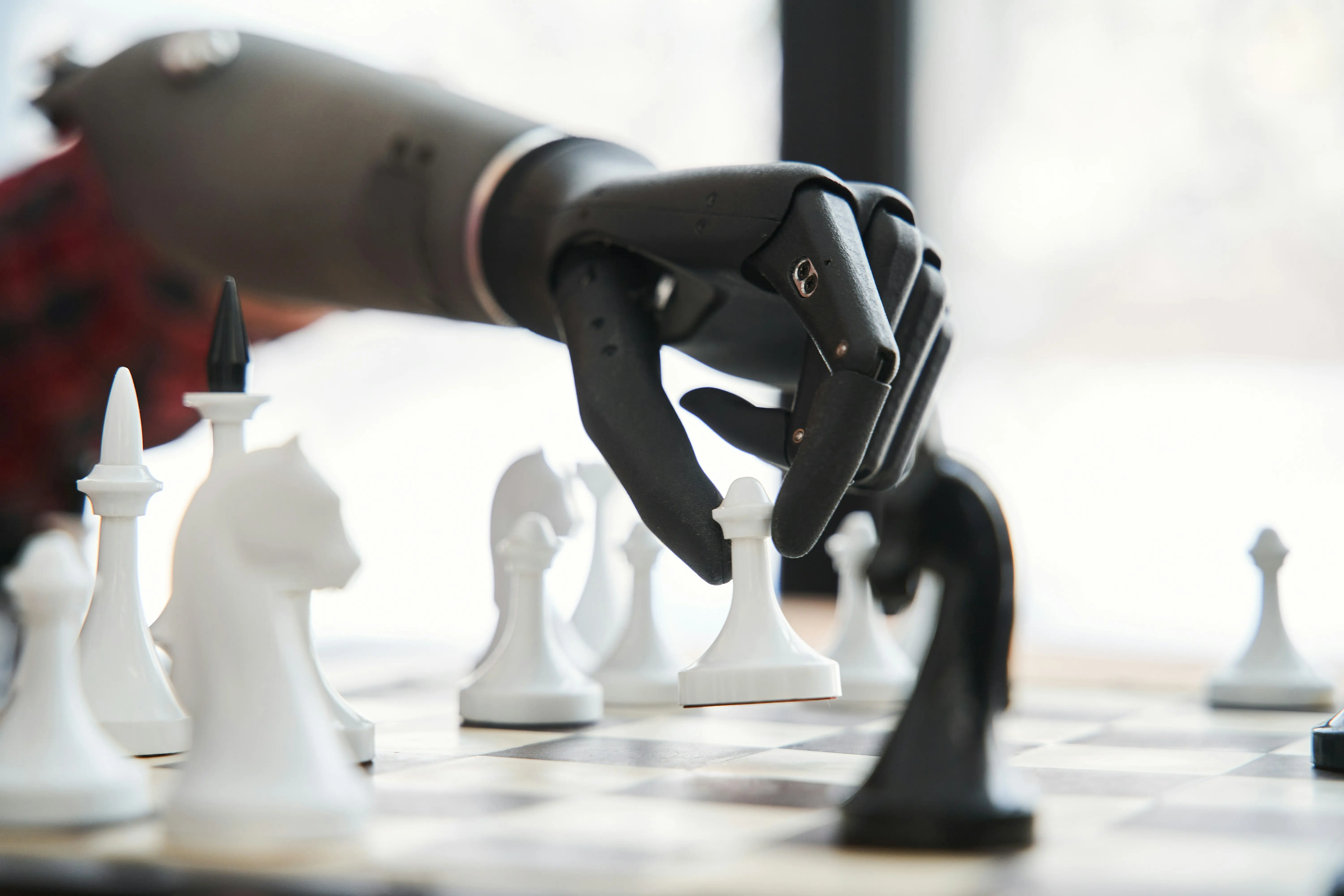
3. Agents d'apprentissage
Un agent apprenant est un agent d'intelligence artificielle qui améliore ses performances au fil du temps en tirant des enseignements de ses expériences.
Il comporte quatre éléments principaux: un élément d'apprentissage, un élément de performance, un critique et un générateur de problèmes.
L'élément de performance choisit les actions, tandis que l'élément d'apprentissage ajuste son comportement en fonction du retour d'information. Le critique évalue le résultat des actions à l'aide d'une norme prédéfinie, et le générateur de problèmes suggère de nouvelles actions à essayer pour un meilleur apprentissage.
Cette structure permet à l'agent de s'adapter aux changements, d'affiner ses stratégies et de fonctionner efficacement, même dans des environnements peu familiers.
Exemples d'agents d'apprentissage
- Un agent d'IA cryptographique qui ajuste les stratégies de négociation en fonction des performances du marché
- Un moteur de recommandation qui s'améliore pour suggérer des produits en fonction du comportement de l'utilisateur
- Un chatbot de soins de santé qui apprend des interactions avec les patients pour améliorer la précision du triage

4. Agents basés sur l'utilité
Un agent basé sur l'utilité est un agent d'intelligence artificielle qui choisit des actions en fonction du résultat qui devrait fournir la valeur globale ou l'"utilité" la plus élevée.
Plutôt que de chercher à atteindre un objectif, cet agent évalue les différents résultats possibles et sélectionne celui qui maximise une fonction d'utilité prédéfinie.
Cela lui permet de gérer des situations où il y a plusieurs façons d'atteindre un objectif, ou lorsqu'il faut faire des compromis. Il doit être capable de comparer les options, de prévoir les conséquences et de classer les résultats en fonction des préférences ou des priorités.
Exemples d'agents basés sur l'utilité
- Un chatbot pour les ventes qui hiérarchise les prospects en fonction de leur probabilité de conversion.
- Un système de négociation d'actions qui équilibre le risque et le rendement afin de maximiser les gains à long terme.
- Un chatbot professionnel qui planifie les réunions pour minimiser les conflits et maximiser la commodité.
5. Agents hiérarchiques
Un agent hiérarchique est un agent d'intelligence artificielle qui organise son processus décisionnel en plusieurs couches ou niveaux, les niveaux supérieurs gérant les objectifs abstraits et les niveaux inférieurs les actions spécifiques.
Cet agent décompose les tâches complexes en sous-tâches plus petites, chaque niveau de la hiérarchie étant responsable d'un champ de décision différent.
Les couches de haut niveau peuvent planifier des stratégies à long terme, tandis que les couches inférieures gèrent les données immédiates des capteurs et les réponses en temps réel. La communication circule entre les couches, ce qui permet à l'agent de coordonner les objectifs généraux avec l'exécution détaillée.
Cette structure facilite la gestion de la complexité et l'adaptation des comportements à différents délais ou priorités.
Exemples d'agents hiérarchiques
- Dans le domaine de la fabrication, un agent de haut niveau planifie le processus d'assemblage tandis que les niveaux inférieurs contrôlent les bras robotisés et la synchronisation.
- Dans une usine intelligente, différentes couches gèrent les programmes de production, la coordination des machines et les opérations physiques
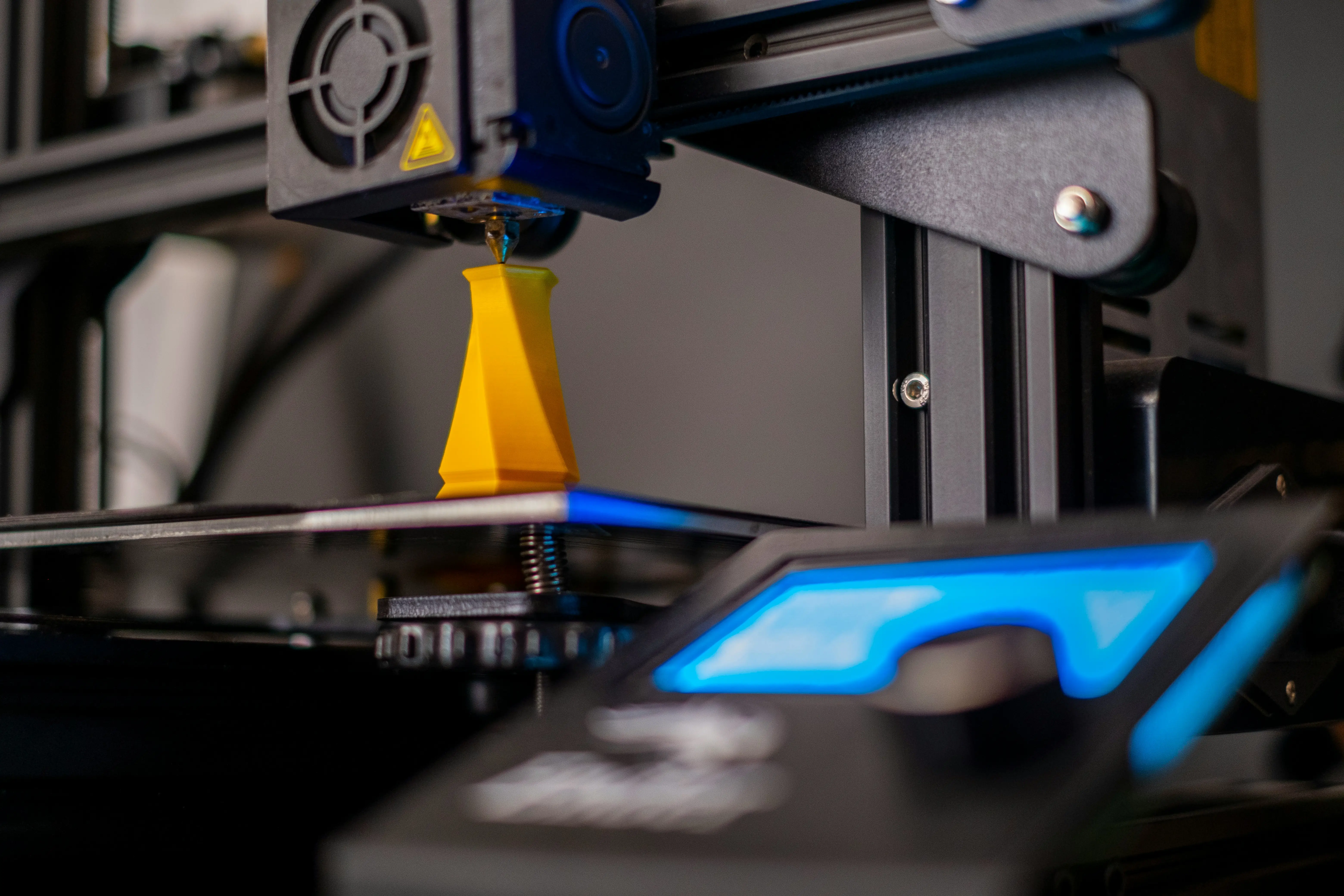
6. Agents basés sur des objectifs
Un agent basé sur des objectifs est un agent d'intelligence artificielle qui prend des décisions en évaluant les actions qui l'aideront à atteindre un objectif spécifique.
L'agent se voit assigner un ou plusieurs objectifs, c'est-à-dire les résultats qu'il souhaite atteindre. Il utilise des algorithmes de recherche ou de planification pour explorer les séquences d'actions possibles, puis sélectionne celles qui sont les plus susceptibles de mener à l'objectif.
Contrairement aux agents réflexes, il ne se contente pas de réagir, il réfléchit aux conséquences futures avant d'agir. Cela le rend plus flexible et plus capable dans des environnements dynamiques ou peu familiers, mais aussi plus exigeant en termes de calcul.
Exemples d'agents basés sur des objectifs
- Un système de navigation qui calcule le meilleur itinéraire pour atteindre une destination.
- Une IA de résolution de puzzle qui recherche les mouvements qui mèneront à la réalisation d'un puzzle.
- Un bras robotisé qui planifie une séquence de mouvements pour assembler un produit avec succès.
7. Systèmes multi-agents (MAS)
Dernier point, mais non des moindres : le système multi-agents.
Un système multi-agents (SMA) est un système composé de plusieurs agents IA en interaction qui travaillent ensemble (ou parfois en concurrence) pour atteindre des objectifs individuels ou partagés.
Chaque agent du système fonctionne de manière indépendante, avec ses propres capacités, ses propres objectifs et sa propre perception de l'environnement.
Ces agents communiquent et se coordonnent, soit directement par des messages, soit indirectement en observant les changements dans l'environnement. Le système dans son ensemble peut résoudre des problèmes qui sont trop complexes ou distribués pour être gérés par un seul agent.
Les systèmes multi-agents peuvent être coopératifs, compétitifs ou un mélange des deux, en fonction de leur conception et de leurs objectifs.
Exemples de systèmes multi-agents
- Des véhicules autonomes se coordonnent à une intersection pour éviter les collisions
- Un ensemble de robots financiers gère la facturation, la détection des fraudes et les rapports grâce à l'automatisation des flux de travail par l'IA.
- Un système de chaîne d'approvisionnement dans lequel différents agents gèrent les stocks, l'expédition et la prévision de la demande.

Créer des agents d'intelligence artificielle personnalisés
Il n'est pas difficile de créer un agent d'IA personnalisé - et vous pouvez le faire gratuitement.
Botpress offre un créateur flux visuel par glisser-déposer, une sécurité de niveau entreprise, une bibliothèque éducative étendue, et une communautéDiscord active de plus de 20 000 créateurs de robots.
Notre plateforme extensible signifie que vous pouvez créer n'importe quel chatbot personnalisé avec n'importe quelle intégration personnalisée - et notre Integration Hub est plein de connecteurs préconstruits vers les plus grands canaux.
Commencez à construire dès aujourd'hui. C'est gratuit.
Questions fréquemment posées
Quels sont les 7 types d'agents d'intelligence artificielle ?
Les 7 types sont : les agents réflexes simples, les agents réflexes basés sur des modèles, les agents basés sur des objectifs, les agents basés sur l'utilité, les agents d'apprentissage, les agents hiérarchiques et les systèmes multi-agents.
ChatGPT est-il un agent d'intelligence artificielle ?
Oui, ChatGPT peut être considéré comme un agent d'intelligence artificielle - il reçoit des données, les traite et génère des réponses, souvent en utilisant une approche axée sur les objectifs ou l'utilité, en fonction de la manière dont il est déployé.
Que sont les agents intelligents et comment fonctionnent-ils dans les environnements numériques ?
Les agents intelligents sont des entités conçues pour agir dans divers environnements numériques. Ils recueillent des connaissances sur leur environnement, évaluent la situation actuelle et exécutent des actions pour atteindre des objectifs prédéfinis. Leurs performances sont influencées par les actions externes qu'ils entreprennent dans des environnements observables.
Qu'est-ce qui constitue la base de connaissances des agents intelligents ?
Les connaissances des agents intelligents comprennent des informations sur l'environnement, des règles prédéfinies et une compréhension fondamentale de la situation actuelle. Ces connaissances constituent la base de leurs processus décisionnels.
Qu'est-ce que l'élément de performance dans le contexte des agents intelligents ?
L'élément de performance des agents intelligents fait référence à leur capacité à atteindre des objectifs et à prendre des décisions qui optimisent leurs actions dans un environnement donné. Il s'agit d'un élément crucial qui détermine l'efficience et l'efficacité de l'agent.
Les agents peuvent-ils opérer dans des structures hiérarchiques ?
Oui, les agents hiérarchiques sont un type d'agent intelligent qui opère à des niveaux structurés. Les agents de haut niveau supervisent la prise de décision générale, tandis que les agents de niveau inférieur s'occupent de tâches spécifiques dans un cadre plus large. Cette structure hiérarchique permet un fonctionnement efficace dans des environnements complexes.
Les agents intelligents fonctionnent-ils avec une intelligence limitée ?
Oui, de nombreux agents intelligents fonctionnent avec une intelligence limitée, ce qui signifie qu'ils ont un champ de connaissances et de capacités défini. Cette limitation les aide à se concentrer sur des tâches et des environnements spécifiques où leur expertise est la plus pertinente.
Comment l'intelligence artificielle joue-t-elle un rôle dans la fonctionnalité des agents ?
L'intelligence artificielle permet aux agents intelligents d'apprendre, de raisonner et de s'adapter. Les agents utilisent l'IA pour améliorer leur base de connaissances, ce qui leur permet de prendre des décisions plus sophistiquées dans divers environnements.